Method for denoising single-cell RNA sequencing data published in Nature Methods
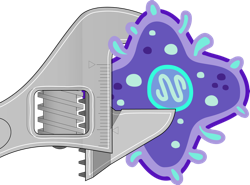
Droplet-based single-cell assays generate systematic background noise with negative impacts, such as off-target gene expression in unexpected cell types and spurious differential expression results. To address this, Stephen Fleming, Mehrtash Babadi, and colleagues developed a deep generative model that distinguishes droplets containing cells from those without, learns the background noise profile, and retrieves uncontaminated counts from cell-containing droplets. They implemented the model in a scalable and robust open-source software package called CellBender and demonstrated the beneficial effects of denoising using simulated data and several real datasets. Read the full paper in Nature Methods and the research briefing here.